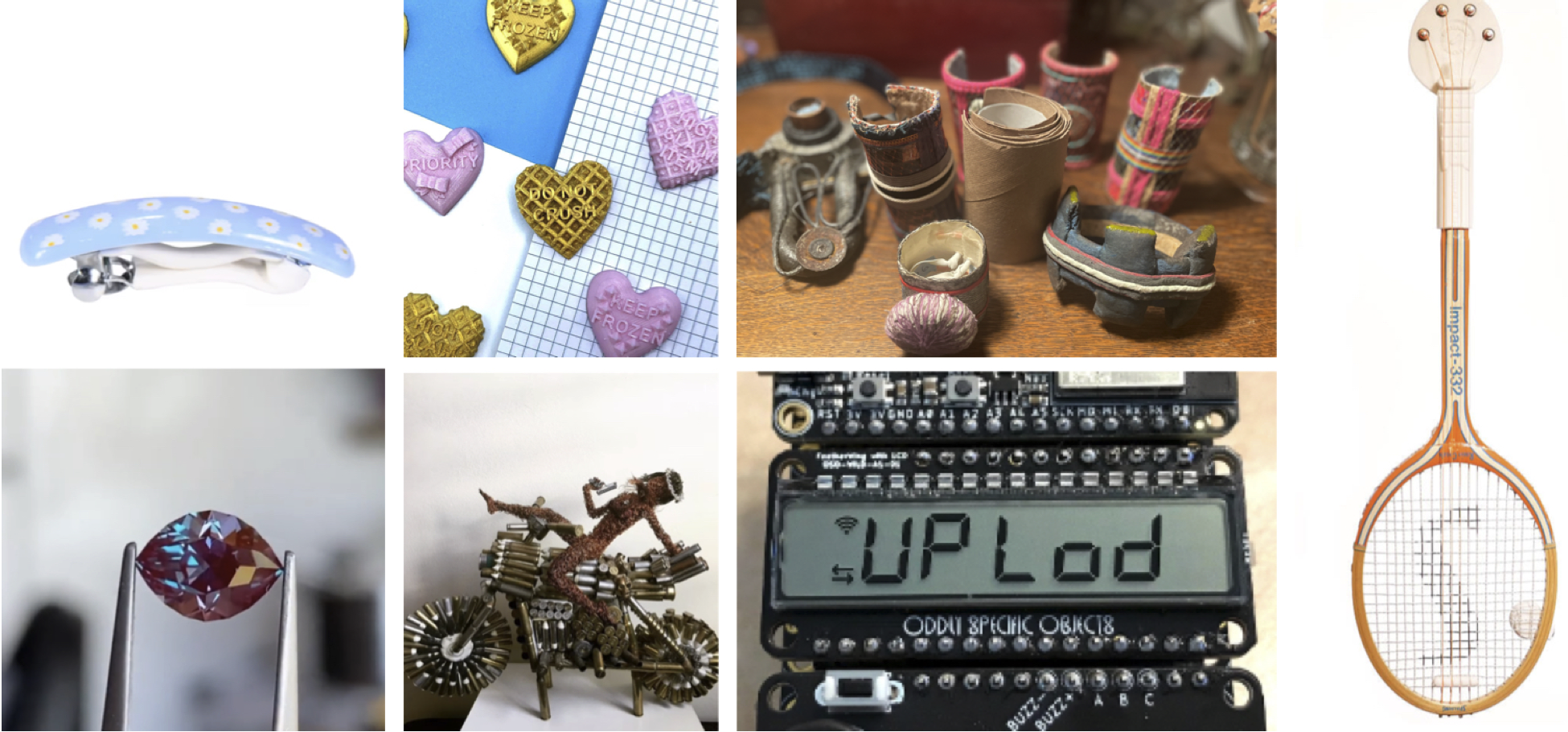
Understanding the Challenges of Maker Entrepreneurship
Jan 2025
The maker movement embodies a resurgence in DIY creation, merging physical craftsmanship and arts with digital technology support. However, mere technological skills and creativity are insufficient for economically and psychologically sustainable practice. By illuminating and smoothing the path from maker to maker entrepreneur, we can help broaden the viability of making as a livelihood. Our research centers on makers who design, produce, and sell physical goods. In this work, we explore the transition to entrepreneurship for these makers and how technology can facilitate this transition online and offline. We present results from interviews with 20 USA-based maker entrepreneurs (i.e., lamps, stickers), six creative service entrepreneurs (i.e., photographers, fabrication), and seven support personnel (i.e., art curator, incubator director). Our findings reveal that many maker entrepreneurs 1) are makers first and entrepreneurs second; 2) struggle with business logistics and learn business skills as they go; and 3) are motivated by non-monetary values. We discuss training and technology-based design implications and opportunities for addressing challenges in developing economically sustainable businesses around making. Find the paper here: https://arxiv.org/abs/2501.13765.
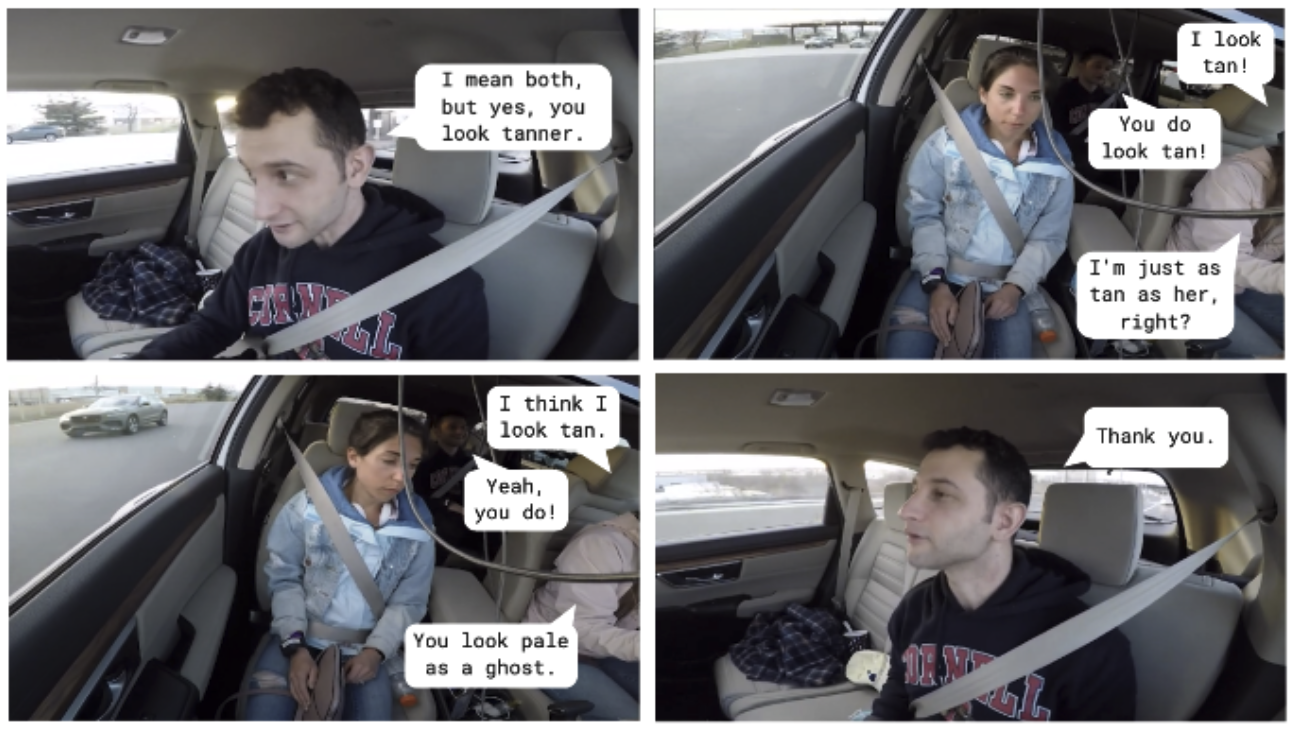
(Social) Trouble on the Road: Understanding and Addressing Social Discomfort in Shared Car Trips
Jan 2024
Unpleasant social interactions on the road can negatively affect driving safety. We recorded nine families going on drives and performed interaction analysis on this data. We define three strategies to address social discomfort: contextual mediation, social mediation, and social support. We discuss considerations for engineering and design, and explore the limitations of current large language models in addressing social discomfort on the road. Read our work here: https://dl.acm.org/doi/10.1145/3640794.3665580
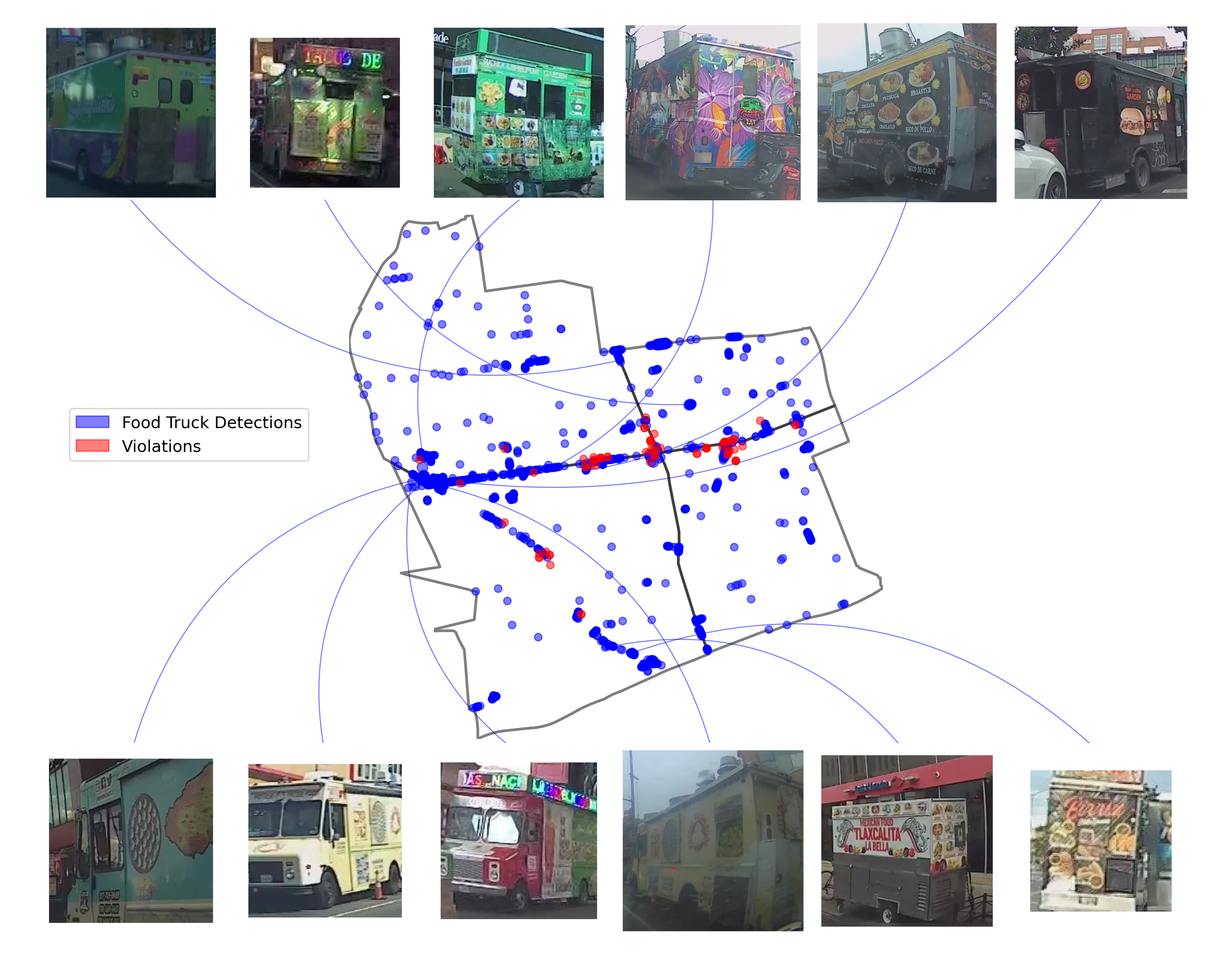
Privacy of Groups in Dense Street Imagery
Jan 2024
Spatially and temporally dense street imagery (DSI) datasets have grown unbounded. In 2024, individual companies possessed around 3 trillion unique images of public streets. DSI data streams are only set to grow as companies like Lyft and Waymo use DSI to train autonomous vehicle algorithms and analyze collisions. Academic researchers leverage DSI to explore novel approaches to urban analysis. To address privacy vulnerabilities, DSI providers have made good-faith efforts to protect individual privacy by blurring sensitive information such as faces and license plates. In this work, however, we find that increased density and innovation in artificial intelligence fail to protect privacy at a group membership level. We perform a penetration test to demonstrate the ease with which group membership inferences can be made from depictions of obfuscated pedestrians in 25,232,608 dashcam images taken in New York City. By synthesizing empirical findings and existing theoretical frameworks, we develop a typology of groups identifiable within DSI and subsequently analyze the privacy implications of information flows pertaining to each group through the lens of contextual integrity. Finally, we discuss actionable recommendations for researchers working with data from DSI providers.
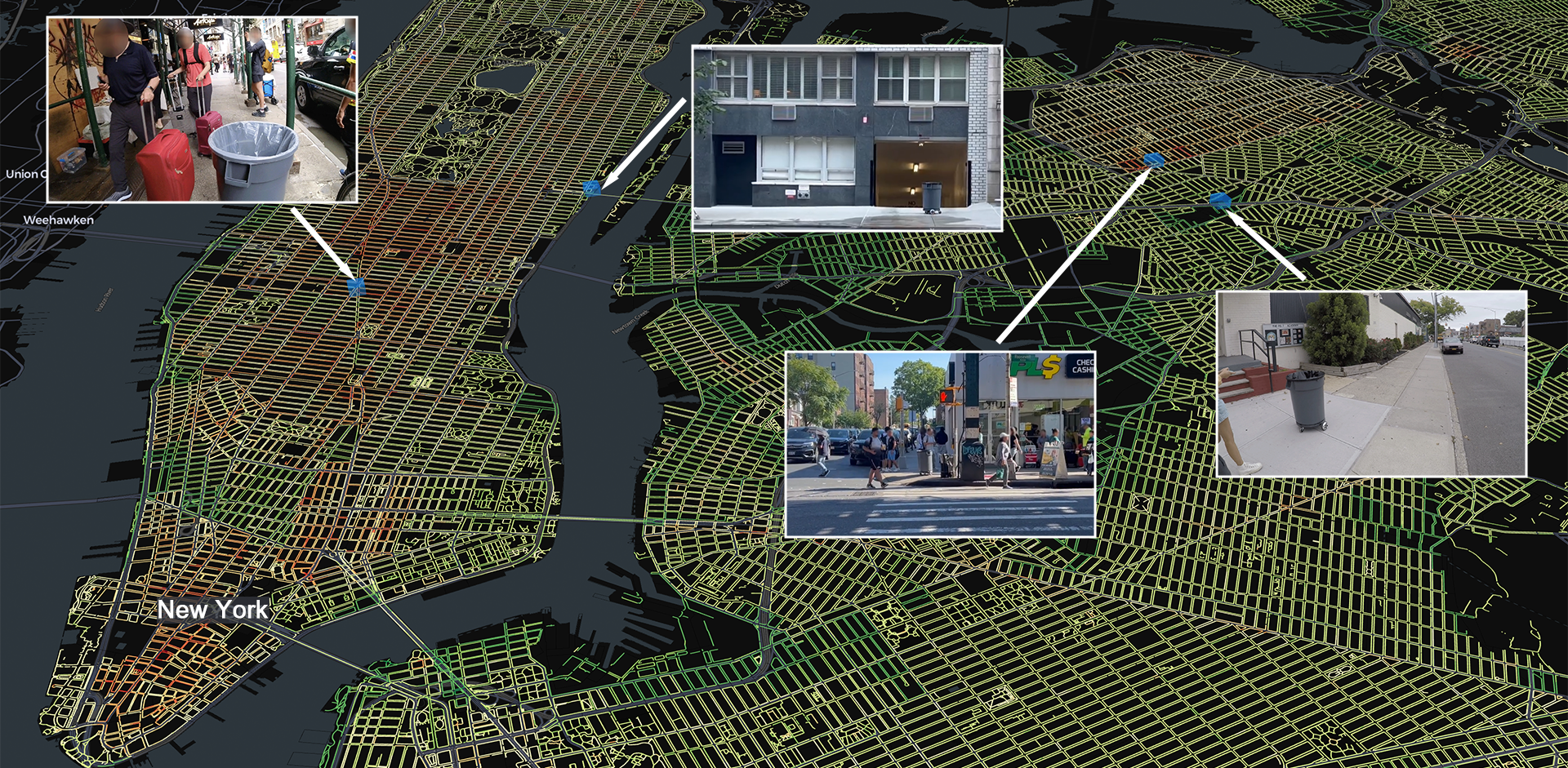
The Robotability Score
Jan 2024
The Robotability Score (R) is a novel metric that quantifies how suitable urban environments are for autonomous robot navigation. Through expert interviews and surveys, we've developed a standardized framework for evaluating urban landscapes to reduce uncertainty in robot deployment while respecting established mobility patterns. Streets with high Robotability are both more navigable for robots and less disruptive to pedestrians. We've constructed a proof-of-concept Robotability Score for New York City using a wealth of open datasets from NYC OpenData, and inferred pedestrian distributions from a dataset of 8 million dashcam images taken around the city in late 2023.
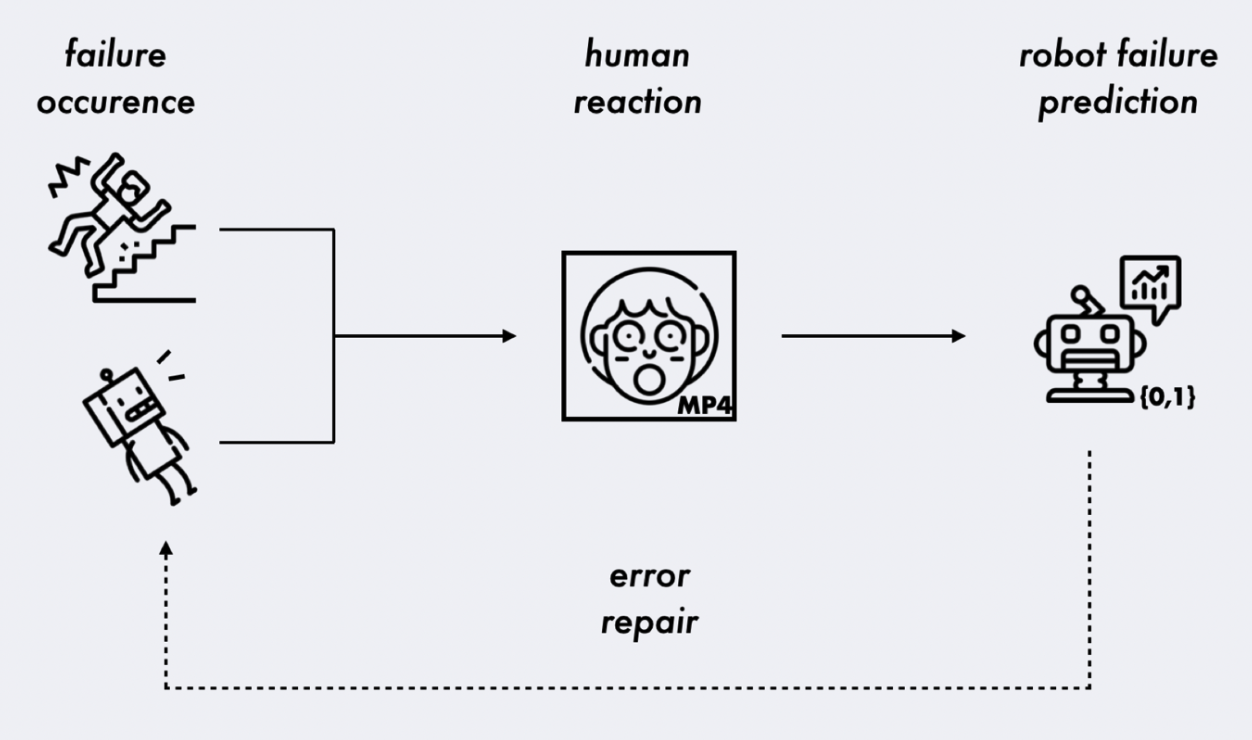
The Bystander Affect Detection (BAD) Dataset for Failure Detection in HRI
Jan 2023
We introduce the Bystander Affect Detection dataset -- a dataset of videos of bystander reactions to videos of failures. This dataset includes 2452 human reactions to failure, collected in contexts that approximate in-the-wild data collection -- including natural variances in webcam quality, lighting, and background. Our video dataset may be requested for use in related research projects. As the dataset contains facial video data of our participants, access can be requested along with the presentation of a research protocol or data use agreement that protects participants. This project is part of a collaborative research effort between Cornell Tech (PI: Associate Professor Wendy Ju) and Accenture Labs. Read our BAD Dataset paper here: https://ieeexplore.ieee.org/document/10342442. Read our larger literature and framework on using social cues to detect task failures here: https://arxiv.org/abs/2301.11972. Request access to the BAD dataset by sending a message through the Qualitative Data Repository (best accessible through Google Chrome): https://data.qdr.syr.edu/dataset.xhtml?persistentId=doi:10.5064/F6TAWBGS.
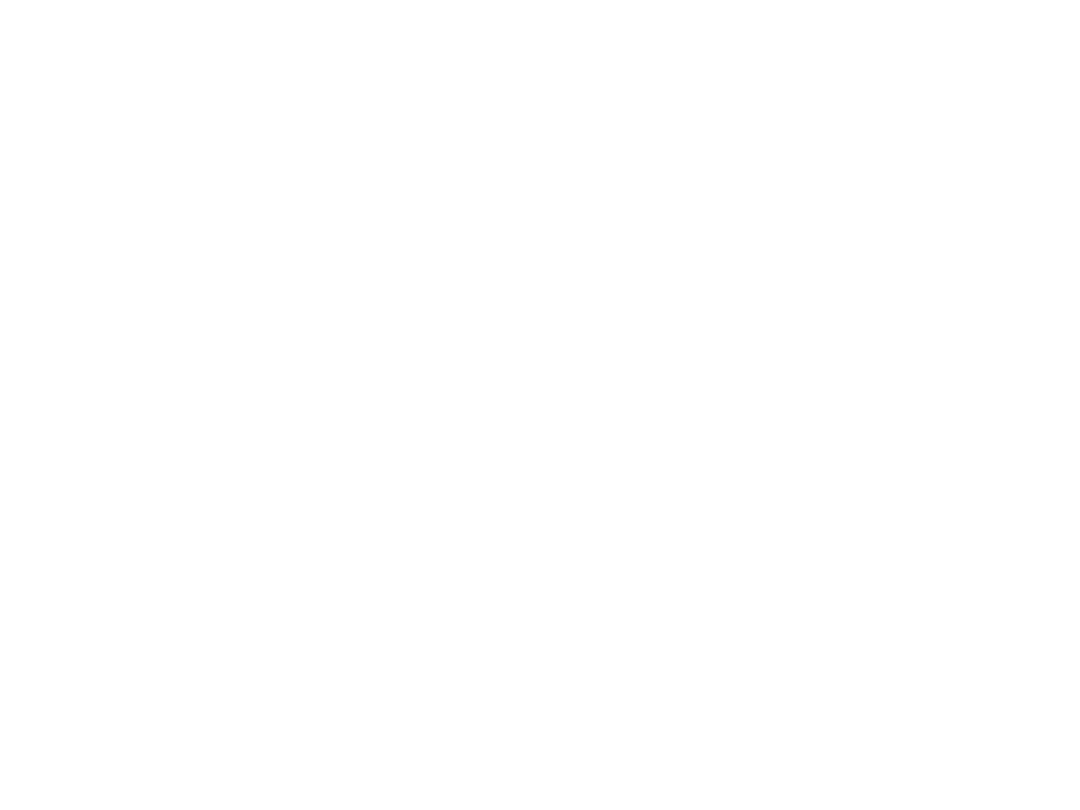
Disparities in police deployments with dashcam data
Jan 2022
Large-scale policing data is vital for detecting inequity in police behavior and policing algorithms. However, one important type of policing data remains largely unavailable within the United States: aggregated police deployment data capturing which neighborhoods have the heaviest police presences. Here we show that disparities in police deployment levels can be quantified by detecting police vehicles in dashcam images of public street scenes. Using a dataset of 24,803,854 dashcam images from rideshare drivers in New York City, we find that police vehicles can be detected with high accuracy (average precision 0.82, AUC 0.99) and identify 233,596 images which contain police vehicles. There is substantial inequality across neighborhoods in police vehicle deployment levels. The neighborhood with the highest deployment levels has almost 20 times higher levels than the neighborhood with the lowest. Two strikingly different types of areas experience high police vehicle deployments — 1) dense, higher-income, commercial areas and 2) lower-income neighborhoods with higher proportions of Black and Hispanic residents. We discuss the implications of these disparities for policing equity and for algorithms trained on policing data.
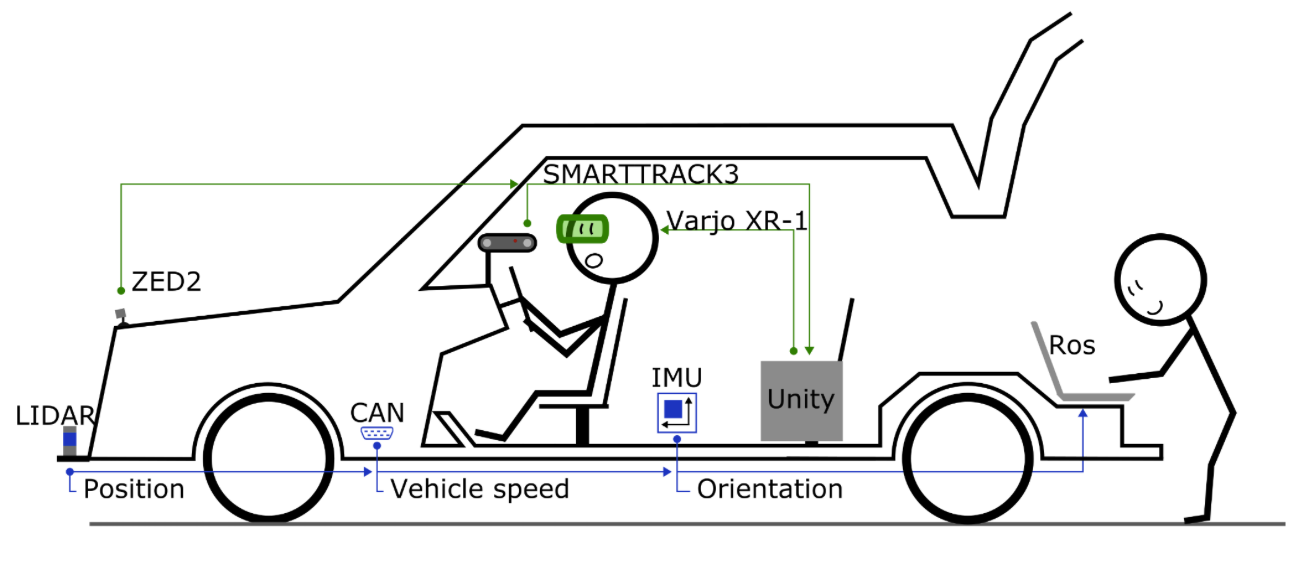
XR-OOM: MiXed Reality Driving Simulation With Real Cars
Jan 2022
High-fidelity driving simulators can act as testbeds for designing in-vehicle interfaces or validating the safety of novel driver assistance features. In this system paper, we develop and validate the safety of a mixed reality driving simulator system that enables us to superimpose virtual objects and events into the view of participants engaging in real-world driving in unmodified vehicles. To this end, we have validated the mixed reality system for basic driver cockpit and low-speed driving tasks, comparing the use of the system with non-headset and with the headset driving conditions, to ensure that participants behave and perform similarly using this system as they would otherwise. Read our paper here: https://doi.org/10.1145/3491102.3517704